Introduction
This article discusses how AI is used to improve the quality of the pictures and videos you can produce.
We chose not to enter the details of how these techniques are working, as we focus on demystifying how AI is currently used to help creators in various ways. However, if you want to learn more about these techniques, you can find links to interesting articles for these different use-cases!
🎨 Note: This article is part of our series on content creation. We hope to provide you with a better idea about the usages of AI for content creators, on a wide range of domains. Don't hesitate to check our other articles!
Image Upscaling
When modifying the size of a picture, we need to remember that the maximum level of detail it contains depends on its number of pixels. It is not valid for vector graphics, but we focus on bitmaps or "classical" pictures in this article.
Suppose you are resizing a 512x512 pixels picture to 128x128 pixels. In that case, there will be 16 times fewer pixels in the final image than in the original one, so information is lost. Suppose you were resizing this 128x128 pixels image back to its original size. In that case, the lost details are not going to be retrieved, and thus the picture looks blurry and not "visually pleasing," as you can see in the image below.
Machine Learning allows us to add details to the picture during the upscaling operation. Using ML, the output looks much better than with a standard upscaling algorithm such as the widely used Lanczos resampling!
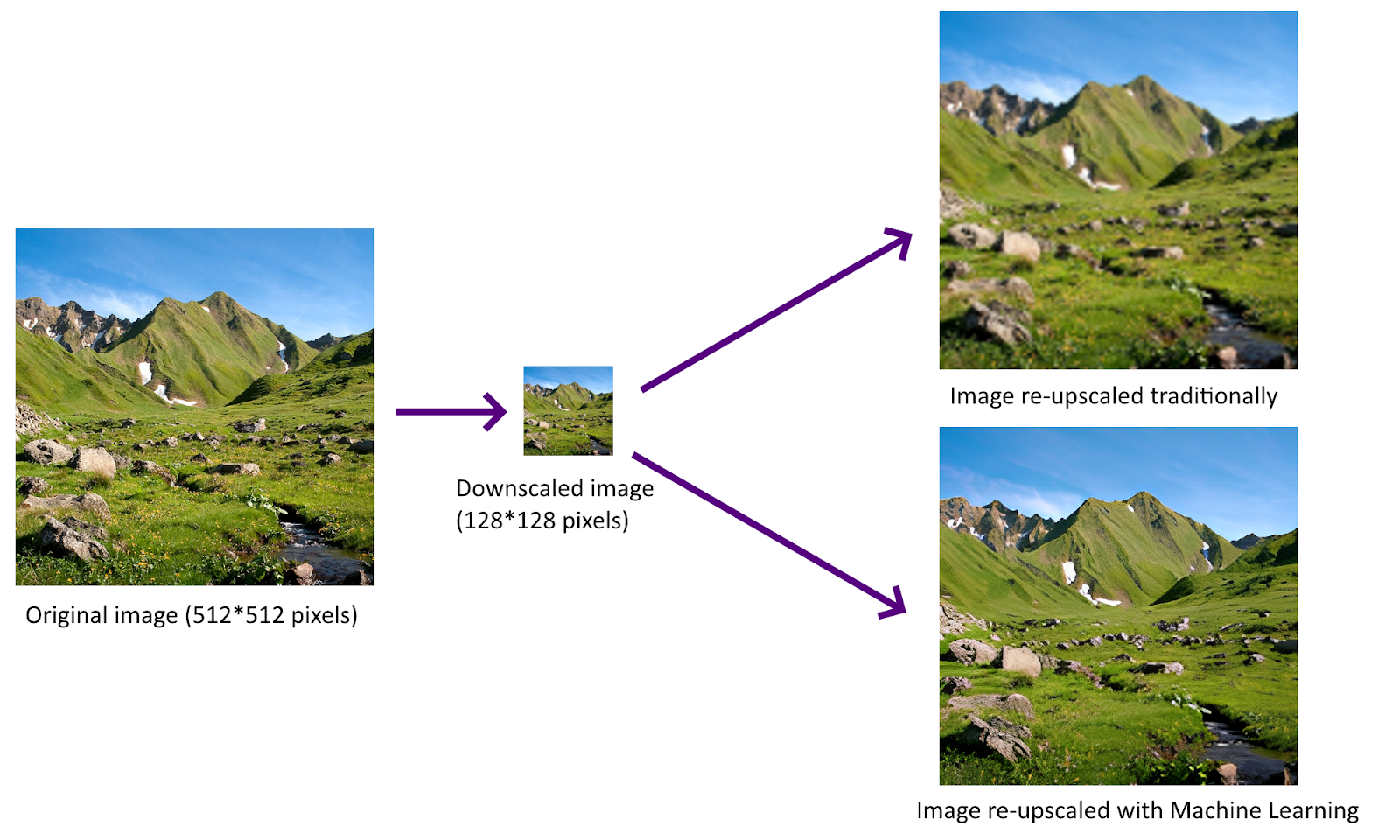
Image upscaling with ML is an effective technique. Still, we need to note that the details created by Machine Learning-based algorithms will not match the original information perfectly. It will only reconstruct as closely to the reality as possible. Only Harry Potter could achieve that.
Sometimes, it also happens that the input image doesn't contain enough information to obtain a convincing result. It is particularly noticeable on faces. Suppose the algorithm doesn't have the information required to recreate a person's distinctive face. In that case, the result will be deceiving and may even be scary!
The information contained in upscaled pictures shouldn't be considered reliable and suitable for running algorithms or studies (like face recognition or medical analysis). Instead, this technique should be used to improve the quality of a beautiful landscape or video game, something that the results do not need to be as close to reality as possible.
Video upscaling and frame interpolation
This technique can also be helpful for videos. However, upscaling a video is more complex than just upscaling each video frame. Indeed, such a process would induce motion and temporal instability issues. Thus, algorithms need to take into account many frames simultaneously to obtain an output with coherent details and where movement looks good.
We can use Machine Learning algorithms to deal with such a challenge. They can easily consider different neighboring frames in a video to produce high-quality results.
The resulting techniques are reliable and widely used. For example, such methods have revived old shows like Star Trek to 4K quality. More details about the final product are available on Star Trek's official website.
To go a step further, we can add more frames to the video by interpolating between the already existing frames. As a result, the video will look “smoother.”
This technique may come particularly handy for hand-made animations, such as stop-motion animations. Here the creator(s) need to take thousands of pictures with infinitely small movements to create a video.
Thus, creating new frames thanks to an algorithm allows artists to save time.
Applications of this technique in the video game industry
We mentioned that we could apply the same technology to video games. When playing a video game, you want to balance the quality of the graphics and the smoothness of the experience. In other words, the user wants to find the optimal resolution where we will maintain a good number of frames per second (fps). Nowadays, most people want to play high framerate games that look just like reality, but achieving this requires a lot of work and heavy processing by the computer or gaming console.
Video-enhancement techniques are used in this context to help create realistic graphics faster. Nvidia and AMD released technologies (DLSS and FSR, respectively) that use Machine Learning to get better images with less processing.
It is less computationally expensive to render each frame at a lower resolution and then upscale them than directly rendering frames at a higher resolution.
You can see this like an inflatable pool. It is much more space and time efficient to deflate it when you are moving it, put it in your backpack, and inflate it back when you arrive than moving the massive thing with five of your friends inside.
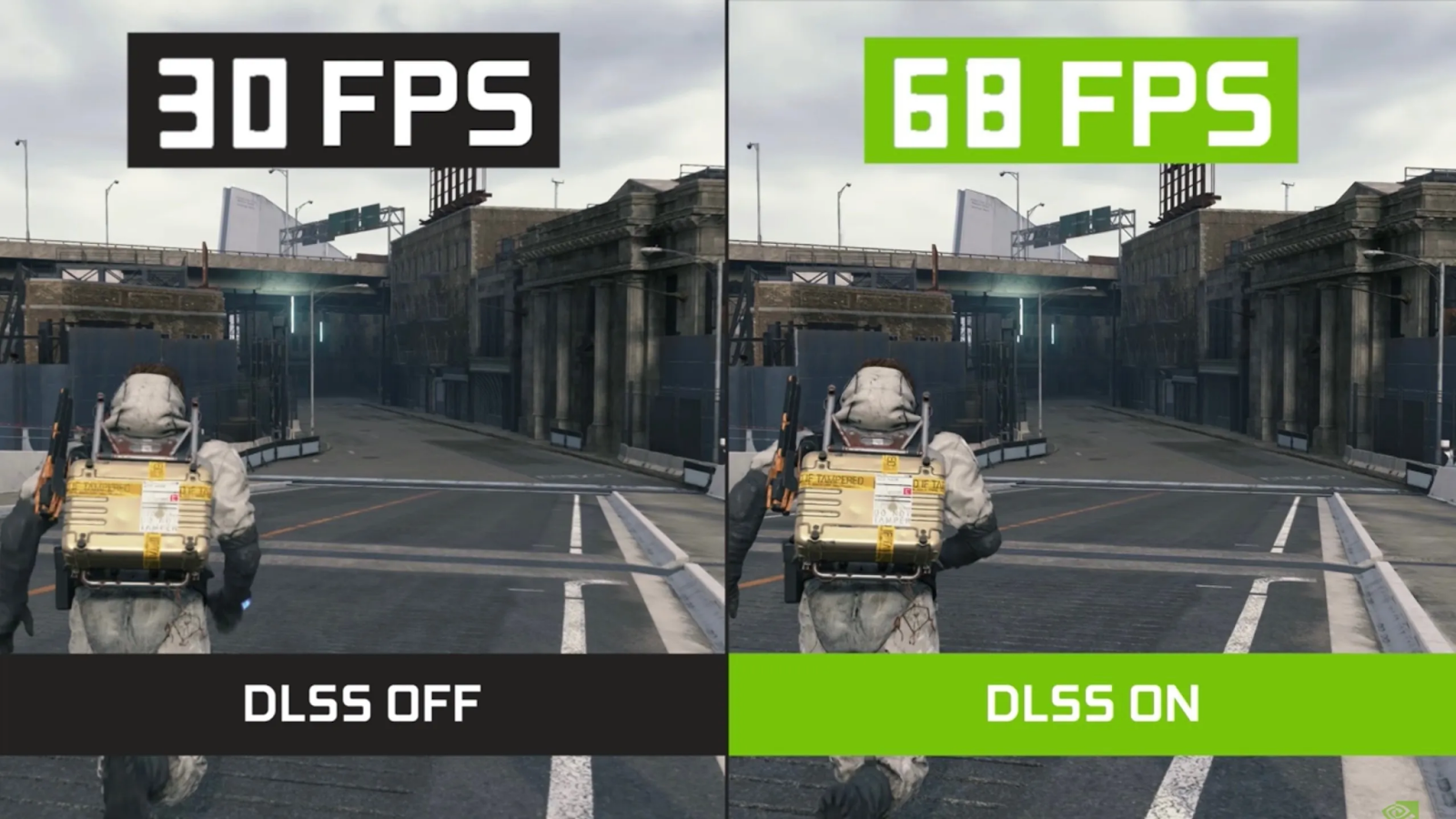
Thanks to this innovative process, we can boost the number of fps and improve the quality of the graphics in no time.
Conclusion
In this article, we discussed the possible applications of AI in the specific techniques of image and video enhancement. We saw how ML could help in the Image Upscaling task and how we can also apply it to video. Frame interpolation is also possible and helps with creators of stop motion animation. Video games are also an area where we can use ML algorithms. DLSS and FSR are technologies developed are video-enhancement tools to get better images with less processing required.
These were some of the most exciting applications of AI we wanted to share, covering image and video enhancement. Still, many more are out there, and many more are to come.
We invite you to read our other articles about content creation. They are highly related to this one using similar algorithms with different data types, like 3D data, sounds, etc., all with the common goal of helping creators.